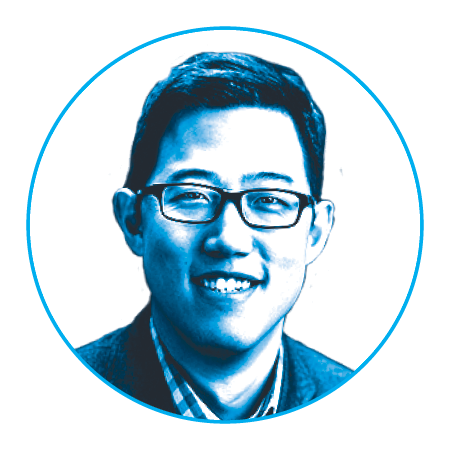
_Edward Kim
Kim is an associate professor in the College of Computing & Informatics.
Imagine staring at a computer screen for days on end without even a millisecond of downtime. Given no other mechanism to modulate the input signal, the neurons in your brain would be continuously forced to fire, eventually spiraling out of control.
In the same way, a team made up of College of Computing & Informatics Associate Professor Edward Kim with researchers from the Los Alamos National Laboratory discovered that simulated neural networks become unstable after continuous periods of processing analogous to unsupervised learning (such as when a system is searching for patterns in a large collection of data).
The researchers found that the systems regained stability when exposed to states that are analogous to slow-wave patterns that human brains experience while sleeping. They created this state by injecting Gaussian noise waves, made of varying frequencies and amplitudes, into the neural network — like turning on AI’s own little white-noise machine.
Their discovery was published in the Proceedings of the IEEE/CVF Conference on Computer Vision and Pattern Recognition (CVPR) Workshops, and was also featured in Popular Mechanics, Discover Magazine and Fortune.
What’s next for the researchers? Implementing their algorithm on Intel’s Loihi neuromorphic chip, which mimics the neural structure of the human brain. By letting the chip catch some metaphorical Zzz’s, it might better process information from a silicon retina camera.