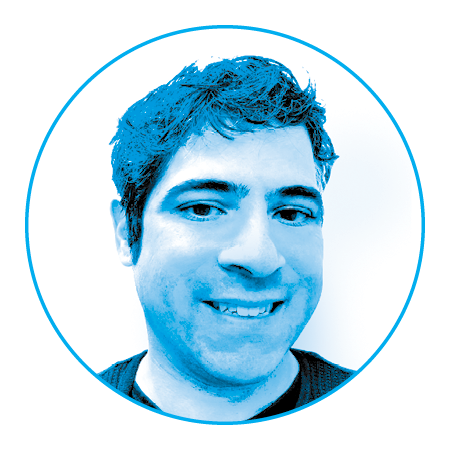
_Bahrad A. Sokhansanj
Sokhansanj is an assistant research professor in the College of Engineering.
Drexel researchers hope to be among the first to spot the signs of the next dangerous COVID variant, thanks to a computer model developed in the College of Engineering.
The model uses machine learning algorithms trained to identify correlations between changes in the genetic sequence of the COVID-19 virus and upticks in transmission, hospitalizations and deaths.
The program can quickly hone in on the areas of the genetic sequence that are most likely to be linked to changes in the severity of the variant.
Some estimates suggest that SARS-CoV-2 has only explored as little as 30–40% of the potential space for spike mutations.
“Our model is more like an early warning system for emerging variants,” says Bahrad A. Sokhansanj, an assistant research professor who led development of the computer model. “When we get a sequence, we can make a prediction about risk of severe disease from a variant before labs run experiments with animal models or cell culture, or before enough people get sick that you can collect epidemiological data.”
Genetic and patient data from the GISAID database — the largest compendium of information on people who have been infected with the coronavirus — were used to train the algorithm.
“Some estimates suggest that SARS-CoV-2 has only explored as little as 30–40% of the potential space for spike mutations,” says Gail Rosen, a professor in the College of Engineering who heads Drexel’s Ecological and Evolutionary Signal-processing and Informatics Laboratory.
“When you consider that each mutation could impact key virus properties, like virulence and immune evasion, it seems vital to be able to quickly identify these variations and understand what they mean for those who are vulnerable to infection,” she says.